






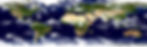


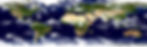
Navigating Analytics
Expertise in Building and Managing Teams, Structures and Processes for Data Management, Analytics, Modeling, Optimization, Business Intelligence Reporting Dashboards and Real-time Streaming from Cloud Technologies

Guidance on Some of the Analytics Needed for the Best in Decision Supports Deliverables to Drive Efficiency, Revenue and Profitability
In Decision Supports Team, certain basic deliverables distinguish this team. These include but not limited to:
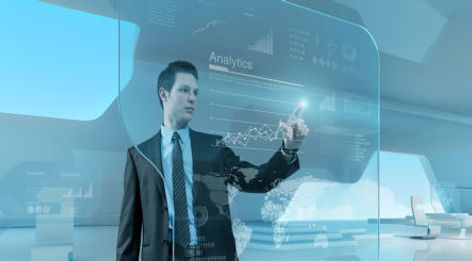


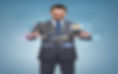

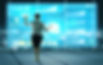
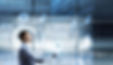
-
Develop and execute analytics strategies designed to facilitate the company’s ability to achieve competitive advantages through the development of proprietary analytics, predictive analytics, fraud analytics and mathematical behavioral models and tools designed to drive higher revenue and profit margins through focused work efforts. Some of the Analytics approaches include among others:
-
Conjoint and Choice Analysis of what the customers want
-
Cluster analysis in market segmentation
-
Adstock modeling in effectiveness of advertising
-
Multidimensional scaling in customers’ idea points
-
Market Basket Analysis and Association Rules
-
OLAP and Data mining
-
The Predictive modeling include among others:
-
Modeling Trend and Seasonality
-
Copernican Principle to Predict Duration of Future Sales
-
Leveraging Neural Networks to Forecast Sales
-
-
The Mathematical based behavioral models include among others:
-
Monte Carlo Simulation and sensitivity analysis in terms of Marketing Decision Making
-
Calculating Lifetime Customer Value
-
Efficient Allocation of Resources in Customer Acquisition and Retention
-
Demand Curves with Solver to Optimize Price
-
Conditional probability in bundling and optimization in variance
-
-
-
Deliver different advanced analytics, risk, statistical, econometrics and mathematical tools, models and methods in areas that include risk management, loss distribution (frequency and severity), scorecard, profit forecast, risk-return matrix, simulation, profit and loss analysis.
-
Leverage appropriate data mining tools (Neural Network, MARSplines, C&RT, CHAID) in business performance and Customer Retention plus advanced family of Regression Models including, Logistic and Multinomial Models (Credit Scoring), Poisson and Negative Binomial Regression (Loss Frequency Prediction) and Log Normal Regression (Severity Prediction). Also, in addition is the use of Design of Experiment (DOE) for Process Design and improvement; and Discrete-event models and simulation for proof of concept, planning, improvement and prediction.
-
Deliver sets of analytics measures, models and thresholds that include:
-
The use of appropriate quantitative tools in determining the correlations between the company-wide risks to know where the leaders can have more leverage to handle the requirements for managing the risks
-
-
Develop mathematical/statistical distributions that are reflected:
-
Risk profiles and Shareholders' value
-
Probability of surplus or/and balance sheet impairment
-
Positive and negative cash flow
-
Extreme losses in catastrophe and non-catastrophe businesses
-
Probability of rating downgrades.
-
-
Establish processes that efficiently identify and quantify wide varieties of risks in terms of trend analysis in areas that include:
-
Portfolio performance
-
Pricing and Reserving
-
Markets and Distribution Channels
-
Customers
-
Underwriting
-
Capacity Management and Volatility Management
-
-
Based on the modeling and analyses, make recommendations to the C-levels on what will be needed to assess risk exposure, reduce or prevent losses (frequency & severity), improve underwriting guidelines, enhance comprehensive risk mitigation, assess capital adequacy, optimize pricing, ensure product-lines profitability and increase market share of wallet in new businesses and renewals.